Cookies are out, and contextual is back in – and the good news is, it’s had an upgrade, says Rosa Almarza, client director at Illuma.
Google’s timeline on removing cookies may seem…flexible… but one thing is certain, since GDPR, cookie-based impressions have become less and less available in the programmatic marketplace.
The online advertising industry has relied heavily upon cookie data for the last 20 years, so this seismic shift has caused huge disruption at many levels. But the truth is that smart advertisers had seen this moment coming for a long time. Cookie-based targeting was always hard to justify from a privacy perspective, plus it’s difficult to scale and relies upon old data.
Change was long overdue and great strides are now being taken in developing cookie-alternatives – other ways to look at audience identity.
Yet some of the most innovative new solutions actually don’t involve identity at all, they are coming from a new fusion between contextual and behavioural targeting, powered by AI.
Contextual re-engineered
Contextual targeting has historically involved buying impressions on pages whose content is deemed relevant to a marketer’s brand or product, as defined by keywords and other metrics within the content or URL. For example, an airline might want to appear against content around city breaks, family holidays or global business centres.
It’s always been seen as a safe, if rather unexciting, option – advertisers can control the context their brand appears within and increase the likelihood of reaching users with the right interests. But the ease of contextual has also held it back – it’s been seen as one-dimensional and limited in scale, and brands can often end up appearing shoulder to shoulder with their direct competitors.
Thanks to recent innovations in AI and machine learning though, the shortcomings of contextual targeting are now being dismantled one by one, and AI-powered contextual targeting is gaining momentum as a strong alternative to cookies.
Here are 3 ways that AI is supercharging contextual targeting.
1) Live and dynamic
Like most targeting, contextual tends to involve buying against static, one-dimensional metrics – for example, topic, keywords, and more recently, things like sentiment, tone and article length. Marketers and traders decide in advance which metrics to pursue in the hope of reaching the brand’s KPIs.
As a campaign unfolds, and traders can see what’s working, they may wish they’d made different decisions. But adapting the targeting on the fly to secure more of those impressions has simply been impossible, due to the speed of data processing required.
However, data processing speeds are now rapidly increasing thanks to AI and machine learning, to the point of enabling a live, dynamic feedback loop to contextual targeting. This means updating the URLs based on what is working best, while a campaign is running. Applying AI in this way is having a considerable impact on campaign outcomes.
2) Relevance with scale
When live engagement signals and attention clusters are used as a data input, rather than just pre-set metrics, a campaign can be ‘freed’ from these limits. Targeting still takes place within a brand-safe framework, but rather than narrowing into contextual corners it expands across a range of topics to find new and unique audiences based on these live data signals – in predictable and unpredictable places.
Driven by engagements and attention metrics in this way, a campaign can scale intelligently and explore an almost endless number of quality contexts without diluting audience relevance. This relevant scale is also making a significant difference to campaign results.
3) All of the insights, all of the time
Audience modelling based on contextual consumption is a common way for brands to expand campaigns. It involves matching first-party IDs to content URLs in the bid stream – effectively taking a snapshot of customers’ contextual interests in order to add an additional line to the targeting.
The next step is for contextual modelling to keep pace with first-party users throughout the campaign – not just in that one moment in time. AI and machine learning are making this possible, and the huge increase in input data is resulting in a significant expansion in relevant new audiences.
For example, a campaign line may focus on six or seven contextual topics when a first-party audience is considered once, but 60 or 70 topics when it’s allowed to mirror the audience fluidly throughout the campaign. Again, this contributes hugely to both relevance and scale.
In summary, contextual targeting has always been one-dimensional and involved marketers and traders predicting the contexts that might work. The targeting couldn’t dynamically adapt because there was no way to process the data fast enough. Developments in AI have changed this, and as a result, AI-powered contextual targeting is now audience-led, and able to track and model content consumption behaviour in real time, honing in on live audience relevance and campaign performance in a way that’s not been seen before.
With these new powers, contextual targeting is not only offering an alternative to cookies, it’s out-performing them on any number of KPIs. Cookies may be in their final months, but with AI supporting a new generation of tools and solutions, eventually I doubt too many people will mind.
Originally published in Spanish on El Programa de la Publicidad 28/07/2023.
To learn more about Illuma’s AI-powered contextual solutions, click here.
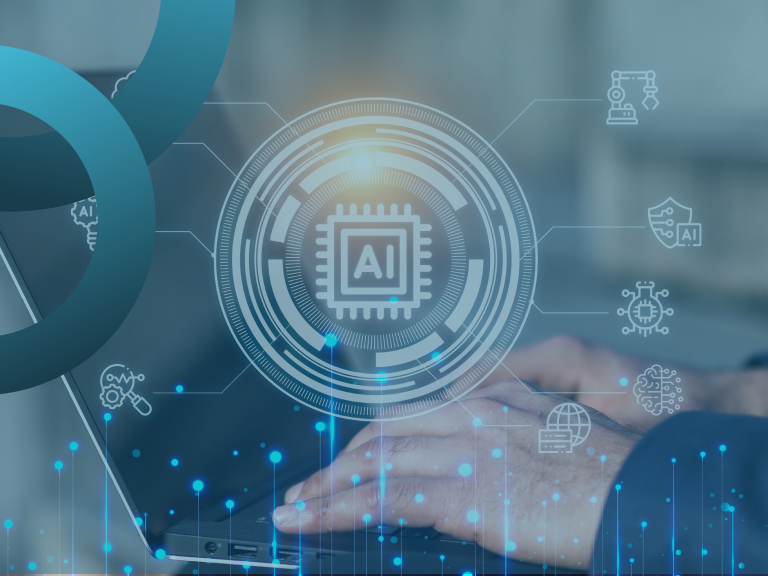